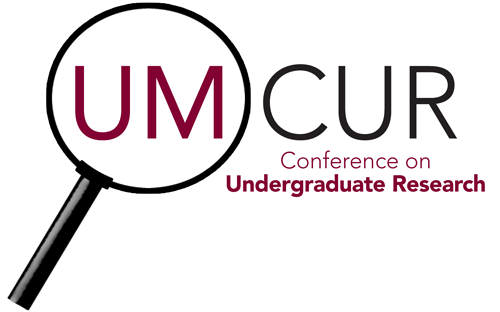
Oral Presentations - Session 3E: UC 332
Modeling the RNA-Nucleocapsid Protein Interactions of Rift Valley Fever Virus Using a Combined Bioinformatics/Biochemical Approach
Presentation Type
Presentation
Faculty Mentor’s Full Name
Steve Lodmell
Faculty Mentor’s Department
Chemistry & Biochemistry
Abstract / Artist's Statement
*Note: Jeremy St. Goddard will be joining Alec during this presentation. Jeremy did the computational work that led to the biochemical work of this project.
Rift Valley fever is the result of a dangerous virus that infects both humans and livestock and shows potential for global spread. To this end, we are interested in disrupting the interaction of viral nucleocapsid protein, N, with the viral RNA genome, that is necessary for replication. This knowledge could lead to the development of new antiviral therapies. The first steps require an understanding of how the protein recognizes and binds the RNA. We will achieve this via a unique approach using the predictive power of computational science to select novel RNA sequences, or aptamers, that will be synthesized and analyzed for binding to N.
We first programmed an algorithm to look for common features on known N-binding RNA sequences. The core of the algorithm is formed from thermodynamic structure calculations performed by software called FoldAlign; our program integrated and interpreted FoldAlign. We then screened a randomly generated RNA sequence library in silico against the known N-binding RNA sequences. Furthermore, we built a filter within the algorithm to select the highest scoring sequences, as given to us by thermodynamic similarity. These ‘winning’ sequences were then synthesized in the laboratory and their binding properties determined. The relative binding affinities were then compared to the strength of the computational score to determine whether the algorithm was a good predictor of RNA sequences/structures that were recognized in a biochemical assay.
Information from these binding assays will be used to improve the algorithm by integrating in silico sequences that bind well to N protein into the pool of known N-binding RNA sequences, so that we are continually refining the thermodynamic motif(s) that the algorithm looks for. We hope this experiment leads not only to a better understanding of the virus, but also to antiviral therapies that end the deadly and infectious nature of this disease.
Modeling the RNA-Nucleocapsid Protein Interactions of Rift Valley Fever Virus Using a Combined Bioinformatics/Biochemical Approach
UC 332
*Note: Jeremy St. Goddard will be joining Alec during this presentation. Jeremy did the computational work that led to the biochemical work of this project.
Rift Valley fever is the result of a dangerous virus that infects both humans and livestock and shows potential for global spread. To this end, we are interested in disrupting the interaction of viral nucleocapsid protein, N, with the viral RNA genome, that is necessary for replication. This knowledge could lead to the development of new antiviral therapies. The first steps require an understanding of how the protein recognizes and binds the RNA. We will achieve this via a unique approach using the predictive power of computational science to select novel RNA sequences, or aptamers, that will be synthesized and analyzed for binding to N.
We first programmed an algorithm to look for common features on known N-binding RNA sequences. The core of the algorithm is formed from thermodynamic structure calculations performed by software called FoldAlign; our program integrated and interpreted FoldAlign. We then screened a randomly generated RNA sequence library in silico against the known N-binding RNA sequences. Furthermore, we built a filter within the algorithm to select the highest scoring sequences, as given to us by thermodynamic similarity. These ‘winning’ sequences were then synthesized in the laboratory and their binding properties determined. The relative binding affinities were then compared to the strength of the computational score to determine whether the algorithm was a good predictor of RNA sequences/structures that were recognized in a biochemical assay.
Information from these binding assays will be used to improve the algorithm by integrating in silico sequences that bind well to N protein into the pool of known N-binding RNA sequences, so that we are continually refining the thermodynamic motif(s) that the algorithm looks for. We hope this experiment leads not only to a better understanding of the virus, but also to antiviral therapies that end the deadly and infectious nature of this disease.