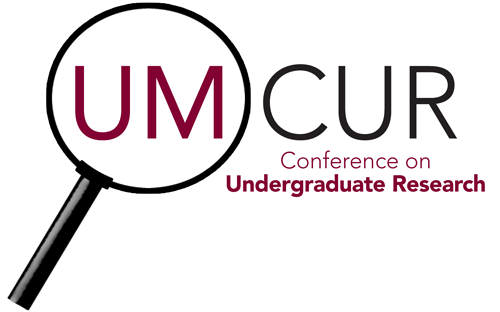
Poster Session #2
Presentation Type
Poster
Faculty Mentor’s Full Name
Dr. Mark Grimes
Faculty Mentor’s Department
DBS
Abstract / Artist's Statement
In statistical clustering, proteins that cluster together are likely to possess a functional relationship with each other. By statistically clustering and filtering proteomic data, networks can be created so that the vast perplexity of protein-protein interaction data can be understood and meaningfully analyzed. Here, glioblastoma and glioblastoma multiforme phosphorylation data was obtained from PhosphoSitePlus and subsequently analyzed using R. The binary data were input into a dataframe and collapsed by their gene names. The Spearman-Euclidean and Euclidean distances were then calculated, with t-stochastic neighbor embedding being performed separately on the outputs. The results were then divided into discrete clusters. Offensively large clusters were broken down to a manageable size via a penalized matrix decomposition. The rank of the penalized matrix decomposition was determined by interpolating values of the data cluster using DINEOF, running PCA on the populated dataframe, plotting the number of principle components against the proportion of variance explained, and finally choosing the point of diminishing returns that still explained over 90% of the variance. Clusters were transformed into network and then visualized in Cytoscape. The final networks represent a useful tool for researchers concerned with protein-protein interactions in glioblastomas. Work is being done to integrate these networks with those obtained from mass spectrometry peak intensities, allowing meaningful analysis of legacy datasets.
Category
Life Sciences
Statistical Clustering of Glioblastoma Multiforme for Graph Theory Analysis
UC South Ballroom
In statistical clustering, proteins that cluster together are likely to possess a functional relationship with each other. By statistically clustering and filtering proteomic data, networks can be created so that the vast perplexity of protein-protein interaction data can be understood and meaningfully analyzed. Here, glioblastoma and glioblastoma multiforme phosphorylation data was obtained from PhosphoSitePlus and subsequently analyzed using R. The binary data were input into a dataframe and collapsed by their gene names. The Spearman-Euclidean and Euclidean distances were then calculated, with t-stochastic neighbor embedding being performed separately on the outputs. The results were then divided into discrete clusters. Offensively large clusters were broken down to a manageable size via a penalized matrix decomposition. The rank of the penalized matrix decomposition was determined by interpolating values of the data cluster using DINEOF, running PCA on the populated dataframe, plotting the number of principle components against the proportion of variance explained, and finally choosing the point of diminishing returns that still explained over 90% of the variance. Clusters were transformed into network and then visualized in Cytoscape. The final networks represent a useful tool for researchers concerned with protein-protein interactions in glioblastomas. Work is being done to integrate these networks with those obtained from mass spectrometry peak intensities, allowing meaningful analysis of legacy datasets.