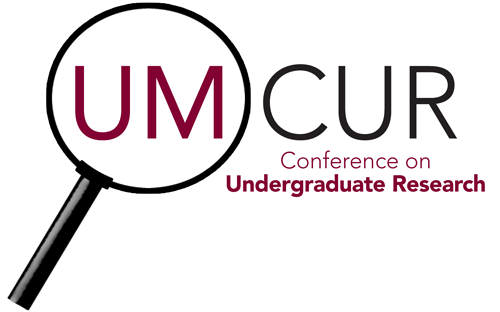
Oral Presentations - Session 1B: UC 327
COMPUTATIONAL PRESERVATION OF THE BLACKFEET LANGUAGE USING MACHINE LEARNING ALGORITHMS
Presentation Type
Presentation
Faculty Mentor’s Full Name
Min Chen
Faculty Mentor’s Department
Computer Science
Abstract / Artist's Statement
Through investigating the audio features of sounds and different machine learning algorithms, we aim to develop a computational framework that automatically identifies and extracts desired sounds from audio clips of the Blackfeet language. The data acquired from this framework will be used to compile a database that will facilitate the digital preservation of the language. Many machine learning algorithms require training data to learn from, and test data to apply that knowledge on. The first step of this project was to create training data by manually identifying occurrences of a desired sound and associating them with sets of quantitative sound features. The next step is to identify a set of audio features that best characterizes the desired sound. This is accomplished through understanding and applying related research results, manual analysis of the training data, and trial and error. The quality of characterization is measured by the percentage of correctly characterized sounds, given a set of audio features and a learning algorithm. This is the first computational linguistic system applied to the Blackfeet language. If successful, similar systems can be implemented for other indigenous languages. Blackfeet is a local Montanan, Native American language that is critically endangered with only 5000 speakers in Canada and 100 in US, most of whom are elderly. Therefore, it is vitally important to preserve this language.
Category
Physical Sciences
COMPUTATIONAL PRESERVATION OF THE BLACKFEET LANGUAGE USING MACHINE LEARNING ALGORITHMS
UC 327
Through investigating the audio features of sounds and different machine learning algorithms, we aim to develop a computational framework that automatically identifies and extracts desired sounds from audio clips of the Blackfeet language. The data acquired from this framework will be used to compile a database that will facilitate the digital preservation of the language. Many machine learning algorithms require training data to learn from, and test data to apply that knowledge on. The first step of this project was to create training data by manually identifying occurrences of a desired sound and associating them with sets of quantitative sound features. The next step is to identify a set of audio features that best characterizes the desired sound. This is accomplished through understanding and applying related research results, manual analysis of the training data, and trial and error. The quality of characterization is measured by the percentage of correctly characterized sounds, given a set of audio features and a learning algorithm. This is the first computational linguistic system applied to the Blackfeet language. If successful, similar systems can be implemented for other indigenous languages. Blackfeet is a local Montanan, Native American language that is critically endangered with only 5000 speakers in Canada and 100 in US, most of whom are elderly. Therefore, it is vitally important to preserve this language.