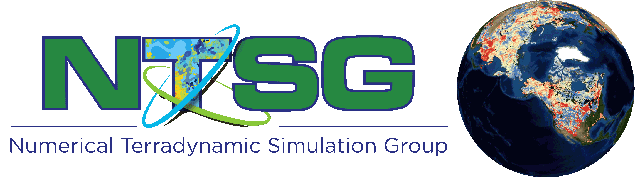
Document Type
Article
Publication Title
Remote Sensing
Publication Date
7-2018
Volume
10
Issue
8
First Page
1
Last Page
17
Abstract
This paper proposes a processing chain for the derivation of global Leaf Area Index (LAI), Fraction of Absorbed Photosynthetically Active Radiation (FAPAR), Fraction Vegetation Cover (FVC), and Canopy water content (CWC) maps from 15-years of MODIS data exploiting the capabilities of the Google Earth Engine (GEE) cloud platform. The retrieval chain is based on a hybrid method inverting the PROSAIL radiative transfer model (RTM) with Random forests (RF) regression. A major feature of this work is the implementation of a retrieval chain exploiting the GEE capabilities using global and climate data records (CDR) of both MODIS surface reflectance and LAI/FAPAR datasets allowing the global estimation of biophysical variables at unprecedented timeliness. We combine a massive global compilation of leaf trait measurements (TRY), which is the baseline for more realistic leaf parametrization for the considered RTM, with large amounts of remote sensing data ingested by GEE. Moreover, the proposed retrieval chain includes the estimation of both FVC and CWC, which are not operationally produced for the MODIS sensor. The derived global estimates are validated over the BELMANIP2.1 sites network by means of an inter-comparison with the MODIS LAI/FAPAR product available in GEE. Overall, the retrieval chain exhibits great consistency with the reference MODIS product (R2" role="presentation">2 = 0.87, RMSE = 0.54 m2" role="presentation">2/m2" role="presentation">2 and ME = 0.03 m2" role="presentation">2/m2" role="presentation">2 in the case of LAI, and R2" role="presentation">2 = 0.92, RMSE = 0.09 and ME = 0.05 in the case of FAPAR). The analysis of the results by land cover type shows the lowest correlations between our retrievals and the MODIS reference estimates (R2" role="presentation">2 = 0.42 and R2" role="presentation">2 = 0.41 for LAI and FAPAR, respectively) for evergreen broadleaf forests. These discrepancies could be attributed mainly to different product definitions according to the literature. The provided results proof that GEE is a suitable high performance processing tool for global biophysical variable retrieval for a wide range of applications.
Keywords
Google Earth Engine; LAI; FVC; FAPAR; CWC; plant traits; random forests; PROSAIL
DOI
https://doi.org/10.3390/rs10081167
Rights
© 2018 by the authors. Licensee MDPI, Basel, Switzerland.
Recommended Citation
Campos-Taberner, M.; Moreno-Martínez, Á.; García-Haro, F.J.; Camps-Valls, G.; Robinson, N.P.; Kattge, J.; Running, S.W. Global Estimation of Biophysical Variables from Google Earth Engine Platform. Remote Sens. 2018, 10, 1167.
Comments
This is an open access article distributed under the Creative Commons Attribution License which permits unrestricted use, distribution, and reproduction in any medium, provided the original work is properly cited. (CC BY 4.0).